Research HighlightsCombining Human and Artificial Intelligence for Input Decisions
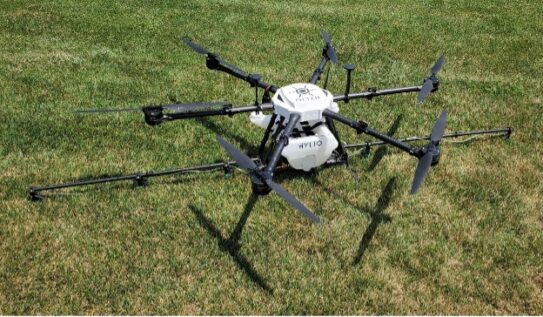
By Laura Temple
Many emerging ag technologies focus on how aerial images obtained with drones can solve problems, observes Scott Shearer, professor and chair of the Department of Food, Agricultural and Biological Engineering at Ohio State University.
“Our research has taken a different approach,” he says. “We explore how drones can extended capabilities of crop scouts. Scouts look inside the crop canopy, especially at the lower leaves, to assess plant health. They aren’t necessarily looking at the upper portion of the canopy from above, like most remote sensing images.”
Shearer’s team started suspending cameras on a 10- to 12-foot pole slung below the drones to see inside the crop canopy more effectively. These drones capture photos as the cameras are inserted into the crop canopy. Those photos are then analyzed to gather information about crop stress.
As technology advances, a camera and a graphical processing unit, or GPU, can be combined to allow for image processing and analysis to happen onboard the drones. With this capability, drones return from field flights with detailed information about crop stress and locations of that stress. Shearer is focusing on using artificial intelligence, or AI, to detect and identify specific types of crop stress.
In soybeans, the Ohio Soybean Council has been funding Shearer’s research focused on weed escapes. In 2022, his team flew drones over research fields, and found that AI correctly identified and mapped the location of key problem weeds: giant ragweed, marestail, Palmer amaranth and waterhemp. They transferred the weed map to a drone equipped to spray inputs to demonstrate the ability to target herbicide application and control those weeds. In actual applications, farmers must observe the pre-harvest interval, allowing the required amount of time between herbicide application and harvest.
“Many weed escapes are resistant to common herbicides,” Shearer says. “Often when farmers notice a few weed escapes, they determine it isn’t worth the effort to load the backpack sprayer and walk out to spray those weeds. However, seeds from those herbicide-resistant weeds can create a serious weed problem in just a couple growing seasons. Based on what we’ve learned, drones offer viable technology to control weed escapes.”
Training Computers with Experts
Key to projects like this one is developing the AI training to get relevant, actionable results from the computer on the drone. For the weed control project, the drone needed to correctly identify problem weeds.
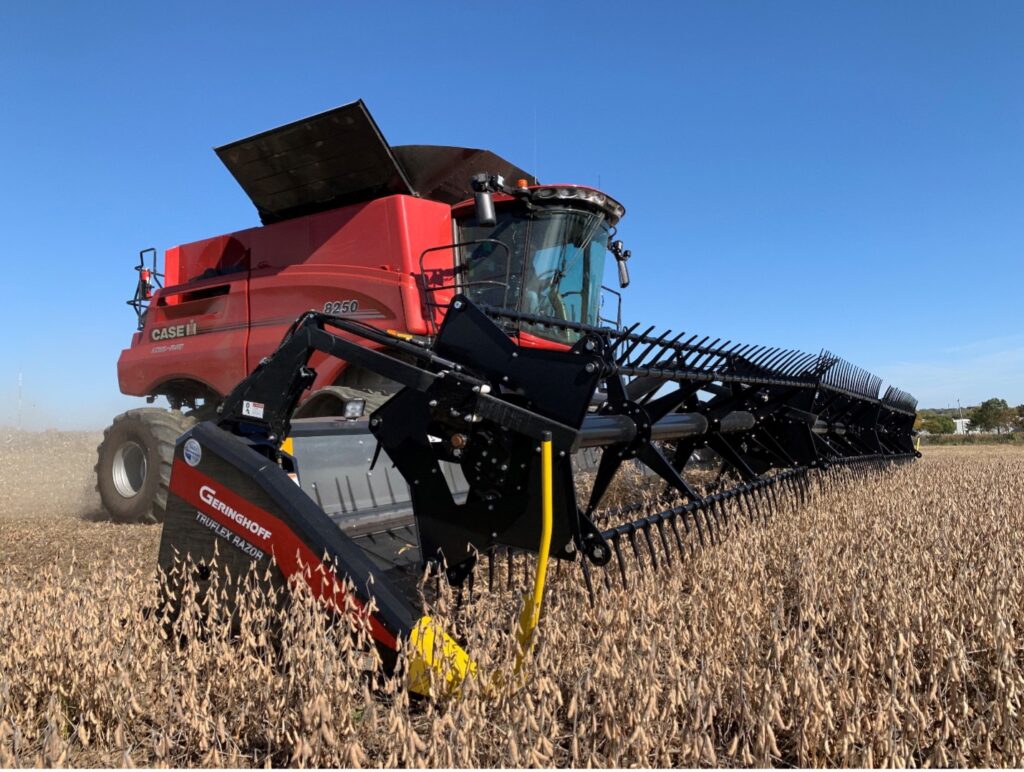
“AI is a black box,” Shearer explains. “We put an image in, and we get a classification in return. Though AI learns quickly, training datasets of millions of images are required. The better the training dataset, the better the performance.”
Shearer’s team worked with Mark Loux, extension state weed specialist for Ohio State University. Loux provided expert input to develop the training image dataset that prepared drones to correctly identify targeted weeds.
Loux recently retired, but Shearer believes that using crop experts to develop the AI use cases and training regiments is like having them ride along on the drone or in the tractor cab to identify problems and help farmers optimize inputs.
“Having these experts guide AI development changes the way we look at crop experts,” he says. “The combination of purposeful AI structure, subject matter experts and computer scientists makes pretty fantastic things possible to help farmers improve their bottom lines while preserving and protecting the environment.”
Shearer believes that including subject matter experts in the AI development and training process can help farmers target crop inputs like herbicides, insecticides and fungicides, to apply only what is economically justified and only where it is needed.
“I think there are 100 or more use cases for this technology for Ohio farmers,” he continues. “My caution to emerging ag technology companies is that they need to involve subject matter experts as they bring products to the marketplace that generate value for farmers.”
Potential AI Applications in Agriculture
“Agriculture adopts technology that makes sense for farmers,” Shearer says. “For example, one of my colleagues recounts multiple companies forming in western Ohio this past summer utilizing drones to apply fungicides to wheat, though we can’t confirm exact acreage. Adding AI may extend the potential benefits of drones in U.S. agriculture.”
Shearer explains that we are just beginning to scratch the surface of how AI will be deployed in agriculture. For example, a camera and GPU can be mounted to spray booms or Y-drops for placing nutrients, managing diseases, controlling insects and more.
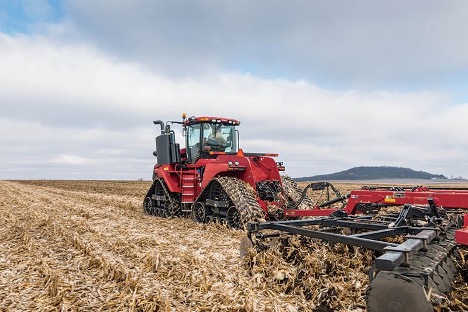
“We have the potential to use AI to essentially put a crop or subject matter expert like Anne Dorrance, professor and soybean disease extension specialist for Ohio State, in the sprayer cab with every soybean farmer,” he says. “That would give Ohio farmers more confidence in the AI-driving application of pesticides. AI can do that with any number of local and regional experts.”
He also thinks it has potential to be used to guide tillage equipment to selectively manage crop residue and soil tilth in preparation for planting.
Each application of the technology requires significant work to develop AI training protocols and datasets to translate images into actionable information. Drones, sprayers and other equipment can act on the AI data to automate management of inputs.
“We are continuing the process of developing a training image database for AI crop stress assessment detection to expand AI applications,” he says. “Various organizations, including the soy checkoff, help fund these efforts, and these datasets are being shared with other groups.”
Leveraging the past and ongoing research support from the soy checkoff created opportunities for Shearer’s team to work with industry on digital agriculture and AI applications. This work prompted the development of a platform to support future AI use case and training dataset development.
Thanks in part to ongoing research and expertise, Ohio State University is leading a multi-institutional, multi-disciplinary AI institute funded by the National Science Foundation. Called the Intelligent Cyberinfrastructure with Computational Learning in the Environment, or ICICLE, the goal is to support the democratization and access of AI in agriculture by building a more accessible cyberinfrastructure. Shearer serves as a team member focused on digital agriculture.
“One challenge ICICLE is addressing is the architecture of where the AI training and processing happens,” he explains. “That processing can be done on the drone, on an edge server, or in the cloud. However, the AI generated by any of these approaches must be made available in the field to drones or sprayers for real-time control of application.”
He believes using integrated human and artificial intelligence will eventually allow farmers to more precisely and profitably manage crop inputs.
Additional Resources:
Using Drones to More Effectively Target Weed Escapes – SRIN article
Published: Feb 6, 2023
The materials on SRIN were funded with checkoff dollars from United Soybean Board and the North Central Soybean Research Program. To find checkoff funded research related to this research highlight or to see other checkoff research projects, please visit the National Soybean Checkoff Research Database.