Research HighlightsUsing Drones to More Effectively Target Weed Escapes
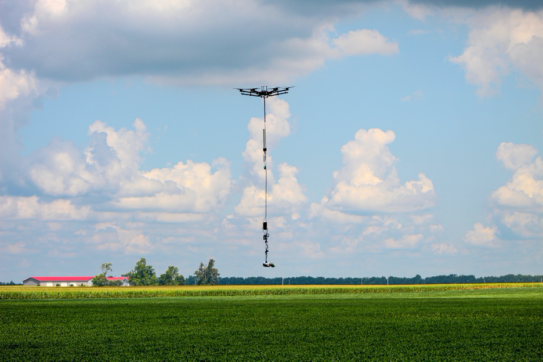
Barb Baylor Anderson
Troublesome weed detection generally involves manual scouting and visual inspection, which can be inefficient, expensive and time consuming. An Ohio Soybean Council checkoff-funded project being conducted at Ohio State University may help soybean farmers map and identify weed escapes with drones (small unmanned aerial systems or sUAS) before targeted eradication.
“To be a farmer today you have to be part scientist, part engineer, part economist,” says Scott Shearer, OSU Food, Agricultural and Biological Engineering chair and principle investigator of the farmer-funded project. “Our goal is to get farmers to adopt this (weed control) technology at the right time that makes the most sense to them and is the most economically viable.”
With drones becoming increasingly available to farmers and with the advances in analytical tools, weed detection and eradication could be accomplished with greater speed and accuracy, says Shearer. Direct benefits of the research will include better nutrient, herbicide and pesticide efficiency, reduction in environmental contamination and a positive impact on the bottom line.
Shearer explains that when humans scout a field, they typically only go to four or five locations within a field, but a drone can visit as many as 400 locations that are uniformly distributed over a field in less time. Drones also are more effective technology than satellite imagery because of the limitations that satellites have, including cloudy days and lower resolution.
The technology allows farmers to discover weeds in earlier stages with a better shot at fixing the problem and containing the weed population for future years. A fiberglass rod is suspended from a multirotor drone down into the plant canopy. The rod has a sensor head with multiple cameras that take photos of emerging weeds. The sensors capture high-resolution imagery, which can be used for real-time plant stress classification.
Photos are taken of weeds escapes in various stages of development and a database of images is compiled to train what is known as Convolutional Neural Networks (CNNs) to identify weeds again in the future. CNNs learn the difference between weeds, dirt, soybeans and shadows to refine their ability to identify the weeds so scouting is more efficient and accurate.
Eventually, Shearer says farmers using the technology could discover plant stressors sooner, allowing them to implement corrective measures earlier and preserve yield and profit potential.
“You would no longer need a selective herbicide – you would no longer need a crop that’s Roundup resistant because you would only be spraying the problem areas,” he says. “You would use as little at 5-10 percent of the typical amount of recommended herbicide. If successful, maybe we won’t need to create herbicide-resistant crops so you’re not broadcast applying over the entire crop. Once perfected, we may view GMOs and herbicides totally differently.”
Shearer asserts the technology will likely start out in the hands of crop advisers who can support implementation costs by helping several farmers. Imagery and data created will also have to be interpreted to actually use them, which is a service that could be provided by an adviser.
Photos courtesy Scott Shearer.
Published: Sep 28, 2020
The materials on SRIN were funded with checkoff dollars from United Soybean Board and the North Central Soybean Research Program. To find checkoff funded research related to this research highlight or to see other checkoff research projects, please visit the National Soybean Checkoff Research Database.