Research HighlightsProgress of a Genomic Selection "Pipeline"
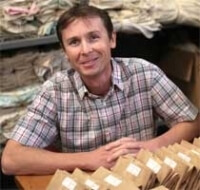
by Aaron Lorenz, Soybean Breeder, University of Minnesota
Increases in soybean yield through breeding have been slower than expected, and much less than that for corn. There are several possible reasons for this, including limited genetic variation in the commercially-used soybean gene pool, the amount of time required for each breeding cycle, the size of the breeding populations, and the accuracy of the evaluations.
The aim of breeding has always been to link phenotype with genotype. Our selections to date are based mainly on phenotype – the yield of a soybean line over many years and locations – so we can be confident that it will perform at that level in farmers’ fields. Because it is both necessary and expensive to generate this field data, we want to put it to maximum use by also collecting genotypic data of the lines and using it in the selection process. Advances in genomics have made whole-genome genotyping less expensive than multi-location yield testing.
A powerful approach to make use of this genomic information for selective breeding is through a method called genomic prediction and selection — the selection of breeding progenies on the basis of predicted performance derived from a statistical model associating genomic information with yield information. The aim of this project, a collaborative effort of 16 soybean breeders in 8 states and funded by the North Central Soybean Research Program, is to develop, test, and make available genomic prediction tools for public soybean breeders in the north central region.
Large datasets of genomic and phenotypic information are required for effective genomic prediction. Fortunately, a wealth of data already exists within the public soybean community. Over the past year, we have collected, cleaned and compiled existing phenotypic, genotypic, pedigree, and environmental data (weather, soil) from decades of yield and diversity tests conducted in the north central region of the U.S.

A primary resource for phenotype data is the historical data from the Uniform Soybean Test, Northern Region — an annual test of the best lines developed by public soybean breeders. The Uniform Tests are divided by maturity group and planted at 5 to 16 locations each. We compiled detailed phenotypic records from 1989-2015 and phenotypic regional summary data from 1941-1988. These data include yield, maturity, lodging, plant height, seed size, seed quality, and various other disease and agronomic screens. The dataset now contains over 7000 soybean lines that have been collectively grown in a total of 1703 environments. In cooperation with all the major soybean breeding companies, extensive yield data has also been gathered on hundreds of high-yielding experimental lines derived from exotic germplasm over more than a decade through research supported by the United Soybean Board.
We are now in the process of collecting seed and genotyping lines in the Uniform Tests. The genotyping by sequencing (GBS) method was chosen for this because it is highly cost-effective. We are also using the Illumina 6K SNP array developed for soybean because it is less technically challenging and is more amenable for use by all soybean breeders and non-experts in genomics and bioinformatics. Future lines entering the Uniform Tests will be genotyped using the 6K SNP array. A secondary but very important goal of this project is to learn how to accurately combine data from these and other genotyping platforms in order to perform a single analysis and create a single predictive model. Combining data from various genotyping platforms is common in human genetics and methods have now been developed for crops.
A relational database scheme has been developed to house the phenotypic data, and we are currently developing an appropriate method to house or directly link to relevant environmental data available from NOAA’s National Centers for Environmental Information, the National Solar Radiation Data Base, and the USDA Soil Survey data. At this point, we have 80% of the weather/soil data compiled. We believe the temperature data is reliable, but the precipitation data is less reliable, so we are looking for better sources of data for the models.
Our next step is to conduct a selection experiment that compares the performance of lines selected using breeders’ current methods to the performance of lines selected using genomic predictions. We believe the development of this methodology is needed for continued genetic gain in soybean, and to bring higher yielding, genetically diverse soybean varieties to farmers. Even with very modest impact on yield, this project could have a large economic impact.
Published: Feb 4, 2017