Research HighlightsDrone Multi-Spectral Imagery Could be Another Tool to Help with SCN Management
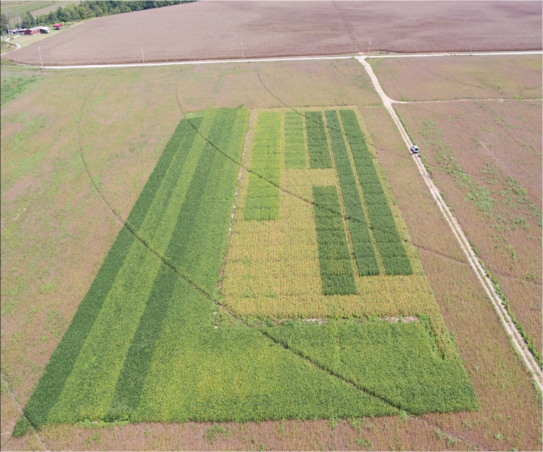
By Carol Brown
The soybean cyst nematode is one of the crop’s most challenging enemies, and scientists across the country are devoted to improving how the soybean plant fights this yield-robbing pest.
Jason Bond, a plant pathologist at Southern Illinois University, has focused his research on nematology and fungi for many years. He is leading a project, funded by the Illinois Soybean Association, that explores another way to support SCN management.
“We know one of the most important aspects of managing soybean cyst nematode is to know what’s going on in the field. The best way to do that is by soil sampling,” Bond says. “But there are limits to this method such as how often should you sample, how many samples per field to collect, and making sure you send the soil samples consistently to the same lab. Doing any of these things differently each time may not provide a true read of what’s happening with SCN in the field.”
Bond is testing unmanned aerial vehicles (UAVs), or drones, to support the recommended practices of soil sampling in the SCN battle. A drone can capture multi-spectral images of plants in a field to reveal levels of plant stress and even potential causes.
“In addition to nematodes feeding on plant roots, there are many things going on in a field to cause plant stress,” Bond explains. “Other pathogens, the environment, soil issues such as compaction, and more, can all stress the soybean plant. Even though they may look lush and green to our eyes, we can expose the plant’s varying stress levels by measuring light reflectance in different bands, or indices.”
Bond and his research team are working to find the right type of UAV imagery to identify plant stress symptoms that are unique to cyst nematodes. UAV image collection is the first and least difficult part of the work, Bond says, while follow-up image analysis is more labor-intensive. The drone captures many images across the entire field, then a computer program stitches the images together. They collect the thousands of images in various light spectrums, including RGB, a common visual spectrum. Bond’s team has found indices other than NDVI (Normalized Difference Vegetation Index) that better relate to SCN infection.
“We want to home in on the signals unique to SCN. The multi-spectral images can give us more information about what’s going on in a field throughout the season,” says Bond. “With the UAV images, producers can get a better handle over years to see how SCN population density changes across the field, as well as what it’s doing to the plant, not just its population in the soil.”
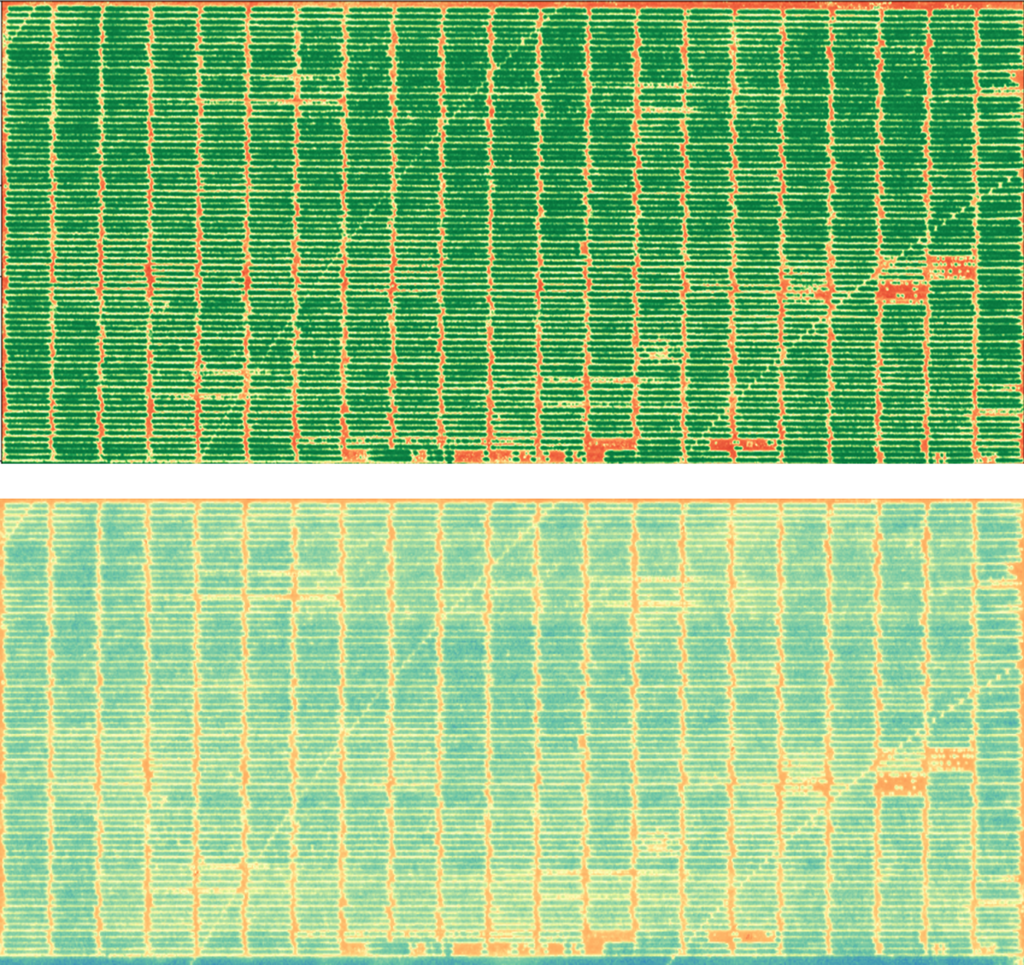
By flying over and capturing field images several times during the growing season, the UAVs can help researchers and farmers learn the true physical stress exhibited on soybean plants. The imagery can also support the sampled soil by confirming or calling attention to problem areas in a field that may be missed by humans.
The team’s “training wheels” for this project stemmed from the remote sensing work on soybean sudden death syndrome, which Bond says, NDVI imagery does an excellent job of detecting and monitoring. They spring boarded from the SDS artificial intelligence models and are adapting them to SCN. His team is working on AI models that will detect stress from SCN and disease levels that go beyond human eye identification.
“There’s a lot to be learned about a field just by the RGB images that commercial drones produce,” says Bond. “Farmers can get real-time information that can’t be gathered by just walking the field. It can be a powerful tool in the toolbox and as the multi-spectral imagery for SCN is perfected, it could become even more powerful.”
A goal of the research project includes developing a workflow to speed up image stitching and separate spectral image indices so they can focus on image analysis. Once these tools are fully validated and made available to the public, industry and consultants could use the computer models to run similar analyses to help farmers in the battle against soybean diseases.
Additional Resources:
IL Soy Advisor video highlights this project
Published: Feb 27, 2023
The materials on SRIN were funded with checkoff dollars from United Soybean Board and the North Central Soybean Research Program. To find checkoff funded research related to this research highlight or to see other checkoff research projects, please visit the National Soybean Checkoff Research Database.