Research HighlightsDigital Image Processing Addresses Consistency of Soybean Grading
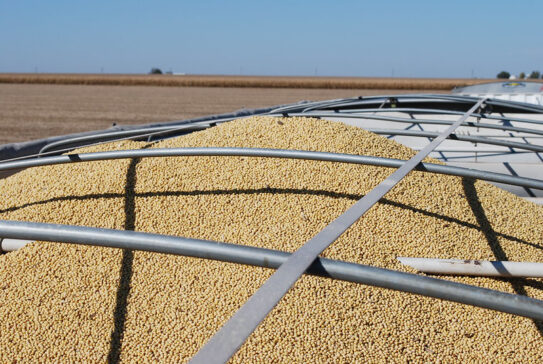
By Laura Temple
Soybeans receive a quality grade of 1 through 5 as they enter the supply chain. That grade has implications for contract fulfillment and the price farmers earn for those soybeans – but that rating can be subjective.
“We heard stories from Louisiana farmers of delivering soybeans to an elevator and believing their soybeans were higher quality than the grade received, so they would go to a different elevator,” explains Kevin Hoffseth, assistant professor in the Department of Biological and Agricultural Engineering, Louisiana State University AgCenter. “One truckload of soybeans could receive three significantly different grades at three different delivery locations. That isn’t efficient for farmers or the soybean supply chain.”
Hoffseth believes digital image processing can provide tools to help grain inspectors grade soybeans more efficiently and consistently. He notes that significant research has been done to use images of soybean plants to address agronomic issues, but much less research has been done on images of soybeans related to inspection and grading. He is leading a research project funded by the Louisiana Soybean and Grain Research and Promotion Board to harness the power of automated computer processing to improve soybean grading.
“People are critical to a process like this, especially to assess factors like smell that don’t show up visually, but the job can become tedious and there is a lot of variability,” Hoffseth says. “Computers don’t care about repetition. You tell them what to do. If an algorithm is set up properly, computers will do the same thing every time, increasing consistency.”
Pictures Allow Visual Analysis
Developing algorithms to tell computers how to assess visual characteristics of soybeans requires looking at a lot of soybeans with both eye and camera. Hoffseth and his team have been laying a foundation to develop and automate this process, incorporating aspects of the visual reference images the USDA provides for grain inspectors.
“Color, shape and texture are the three visual factors that comprise much of the grading process,” Hoffseth says. “We are developing methods to pull quantifiable data on these factors from two-dimensional images.”
Part of developing these initial methods has been figuring out how to standardize the acquisition of soybean images to account for differences in lighting, location and more. If a standard exists, images can be corrected to effectively account for such differences, allowing for consistent analysis.
Hoffseth points out that the USDA visual image references show standard beige soybeans on a non-descript beige background, making it harder for computer processing to differentiate the soybeans from the background. His team developed a custom background plate for soybean photos that increases contrast and has helped more effectively pull data.
Within the images, Hoffseth says computer processing can fit ellipses to individual soybeans and use the resulting geometry to recognize shape and identify the frequency of misshapen beans. Other processes identify textures, like shriveled or wrinkled soybeans, that may indicate frost, weather or other types of damage.
In addition to eventually producing tools that will help grain inspectors ensure consistent, neutral, quantitative assessments of soybean grades, Hoffseth would like to work with the USDA to expand and improve their visual image references for soybean grade.
“Right now, there is just one image for each type of soybean damage,” he says. “While that is valuable, expanding on that set of images would help inspectors. For example, soybean color varies from region to region within the U.S., but that may not impact quality.”
In the future, he expects the data being collected for soybean grading can be used to explore other variability tendencies in soybeans. Images may be able to link tendencies for damage, color and other factors to specific varieties, helping farmers improve variety selection.
In addition to addressing a challenge for the soybean supply chain, Hoffseth explains this project has also had great value for helping engineering students to learn how to extract and analyze valuable information from pictures.
“The applications of image processing are exploding,” he says. “While helping grain inspectors and farmers by making visual assessments of soybeans, my students are learning about the usefulness of digital image processing, and they will be able to apply what they learn to other challenges.”
Published: Aug 2, 2021
The materials on SRIN were funded with checkoff dollars from United Soybean Board and the North Central Soybean Research Program. To find checkoff funded research related to this research highlight or to see other checkoff research projects, please visit the National Soybean Checkoff Research Database.