Research HighlightsCreating a New Breeding Tool Based on Plant Proteins and Machine Learning
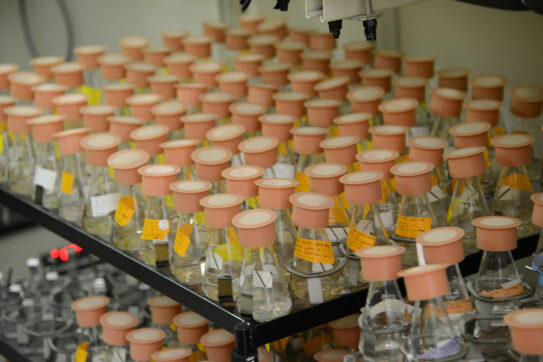
By Laura Temple
How should someone look for a needle in a haystack? Or pick the ripest watermelon in the produce bin?
The right tools, like a fan and a magnet, or the right knowledge, like the sound of a hollow thump, can make a challenging task more manageable.
The odds of finding the needle or choosing the best watermelon may be slim, but those are the odds soybean breeders face regularly as they screen crosses to select the best cultivars to advance. Tools and knowledge like measuring physical traits, or phenotyping, and identifying genetic markers linked to specific traits help them sort through hundreds of potential soybean lines.
Researchers at USDA and North Carolina State University are developing another complementary tool to help soybean breeders select for complex traits, based on plant biochemistry.
“A complex characteristic like heat stress tolerance involves multiple genes, so genetic markers may not be sufficient,” explains plant physiologist Anna Locke, USDA assistant professor at NC State. “And it’s hard to impose consistent environmental conditions on the acres of land used to grow hundreds of genetic crosses for trait comparisons.”
This research is exploring a novel approach to screening for specific characteristics. It involves identifying and measuring protein markers that signal how a plant handles stress, enabled by machine learning-based modeling. The long-term project combines soy checkoff funding from North Carolina Soybean Producers Association and the United Soybean Board with grants from government sources and private companies.
The Biochemical Role of Proteins
Discussing protein in soybeans usually refers to protein content in mature soybeans that becomes soybean meal. But while plants are growing, proteins make things happen at the cellular level.
“Proteins exist in every part of the plant, and they carry out plant functions, like turning carbon dioxide into sugars,” Locke explains. “They send messages and set off reactions that cause plants to turn a gene on or off, and much more.”
At the molecular level, some of that action happens as phosphates, a phosphorus atom attached to a few oxygen atoms, get attached to or detached from proteins. The presence or absence of these phosphate groups directs how plants grow and respond to stress.
“For example, we know that some molecules in plants sense temperature,” she says. “They convey this information and how the plant should respond by adding or removing specific phosphate groups. In the lab, we can measure those phosphate groups on proteins, and use that information to create what we call phosphomarkers.”
Correlating Proteins with Stress Responses
This research has been generating in-depth heat stress data to identify proteins and corresponding phosphomarkers that link to desired heat-tolerance traits. Two lines, one with good heat tolerance and one with poor heat tolerance, were compared under high temperatures both in a controlled growth chamber and then in the field.
“We collected data on genetics, physiological responses, yield, seed content and much more from six growing cycles in the growth chamber and three years in the field,” Locke says.
Leaf tissue samples from the plants can be analyzed with a specific process that measures the attachment of phosphate groups to any type of protein, and then determine what proteins did or didn’t have those groups attached. To measure these protein changes, Locke collaborates with protein sampling expert Ive De Smet at Ghent University, who refined this process.
“Then, with the help of a predictive model using machine learning, we can identify how the quantity of specific proteins and phosphates indicate desired traits for heat tolerance,” she says.
Using Machine Learning to Understand Protein Markers
Locke also collaborates closely with Rosangela Sozzani, professor of plant and microbial biology at NC State. Sozzani is creating the predictive model to analyze protein sampling data and recognize patterns that indicate to heat tolerance.
“When building a predictive neural network, it needs to be interdependent with how the data is collected,” Sozzani says. “Our machine learning model is highly integrated with the way protein samples from soybeans are gathered and processed. Data needs to be curated specifically to answer questions the same and to train the model accurately.”
She is designing the model to identify causal relationships between proteins and heat stress, as well as to correlate protein regulators with plant responses.
“Soybeans are well-defined genetically, but we want the neural network to learn how protein regulators work and how that impacts plant response to heat stress,” she explains.
Sozzani expects machine learning to eventually be able to identify a protein prescription for certain characteristics related to managing heat stress well.
“Once we understand how protein markers reflect phenotypic plant responses, with the help of machine learning, the model should be able to accurately predict how specific varieties will tolerate heat,” she continues. “Then this technology will become very scalable to other characteristics in soybeans and applicable to other crops.”
Selection Support for Soybean Breeders
Locke and Sozzani expect their results will be used to create new tools for breeders to look for protein markers to select the best soybean crosses to focus on when developing new varieties. Using protein markers will complement the way they already use genetic markers and other breeding tools.
“We continue to validate the work we have done so far to increase our confidence in this new method of sorting through soybean lines,” Locke says.
She explains that breeders will either extract proteins from tissue samples and look for identified markers or create a chip that can look for matches to specific markers. Soybean breeders ultimately would be able to test leaves from large quantities of soybean crosses to see if they have the desired phosphomarkers. That information will then be used to determine which varieties should be carried forward to the next stage of soybean breeding.“Breeders will be able to identify potential crosses with strong heat tolerance more quickly and effectively than with tools currently available,” she says.
Additional Resources
Meet the Principal Investigator on this project: Anna Locke
Published: Apr 22, 2024
The materials on SRIN were funded with checkoff dollars from United Soybean Board and the North Central Soybean Research Program. To find checkoff funded research related to this research highlight or to see other checkoff research projects, please visit the National Soybean Checkoff Research Database.