Research HighlightsAutomating Moth Traps to Improve Risk Prediction and Management
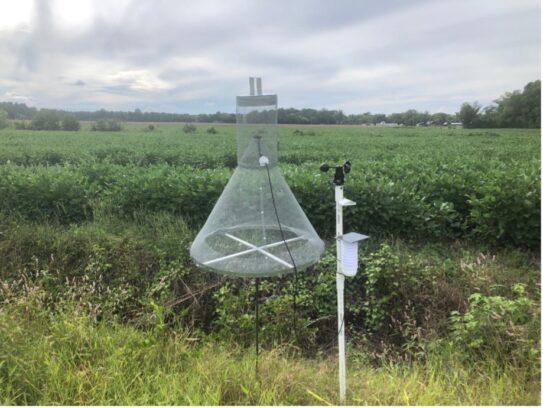
By Laura Temple
For more than 40 years, moth traps have monitored populations of moth pests that feed on soybeans and other crops. Pheromones specific to a moth species attract males to the traps, where they are counted manually. That data informs integrated pest management (IPM) strategies, including local or regional threshold recommendations. This information helps farmers tailor IPM strategies to their fields.
However, the amount of labor, time and resources required to monitor these traps is significant, meaning the data analysis can take days. In North Carolina for example, traps for Helicoverpa zea moths, commonly called corn earworms, are checked every three days, according to Anders Huseth, an assistant professor and Extension specialist at North Carolina State University (NCSU). During that time, moths can reproduce and migrate to new areas, creating another generation that can reduce crop yield.
Huseth is part of a team focused on developing an automated trap that generates real-time, usable data for corn earworm, which is also called soybean podworm, bollworm, tomato fruitworm or something similar, depending on the infested crop.
“With advances in technology, our goal is to develop a platform for inexpensive, adaptable, sensor-based moth traps that can provide real-time information about key soybean pests,” he explains. “This tool will help us predict moth movement and signals farmers can use to improve decision-making for treatment.”
The North Carolina Soybean Producers Association checkoff is supporting this research. Corn earworm feeds on soybean leaves and reproductive tissues. This pest causes significant damage, especially during reproductive stages of soybean growth.
“Soybeans are planted throughout a long window in North Carolina, from late-March through mid-July, and corn earworms infest other key crops,” Huseth says. “Determining when and where corn earworms could become a problem in susceptible soybeans will help prioritize scouting.”
Real-Time Data Gathering and Analysis
The solar-powered traps count the number of moths that pass by an infrared sensor. In addition, they collect environmental data like soil and air temperature, moisture, relative humidity, wind speed and more. Up-to-date information is constantly available for analysis.
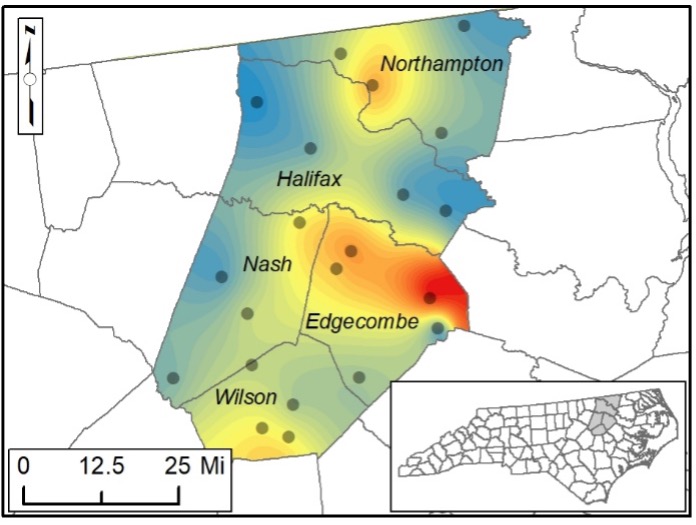
The research team has been continuously refining and deploying improved versions of the automated trap. They developed 10 traps in 2021. In 2022, 15 traps are gathering data for beta testing at an NCSU research farm.
“Our next milestone will be to develop data uplink capabilities, then improve durability and accuracy,” Huseth says. “To manage costs, we have chosen to rely on commercially available components to make the traps.”
The team plans to deploy a network of traps in 2023 and make the data accessible to farmers.
“We believe we can combine data from thesautomated traps with years of historical data to better predict moth flight,” he explains. “One key to this project is figuring out how to convert information from the field into meaningful spatial data maps.”
Using those maps, farmers can focus on scouting in areas where heavy pressure is predicted, especially in fields where crops are at stages most vulnerable to damage and yield loss.
“We want to put the real-time moth count data into context to predict the severity of infestations, and then share it so farmers can decide when and where to treat,” Huseth says. “This information will also show where farmers can save insecticide applications and reduce inputs and costs.”
The data analysis will initially be available on an NCSU Extension website. However, Huseth says a very long-term goal will be sharing the data on more platforms.
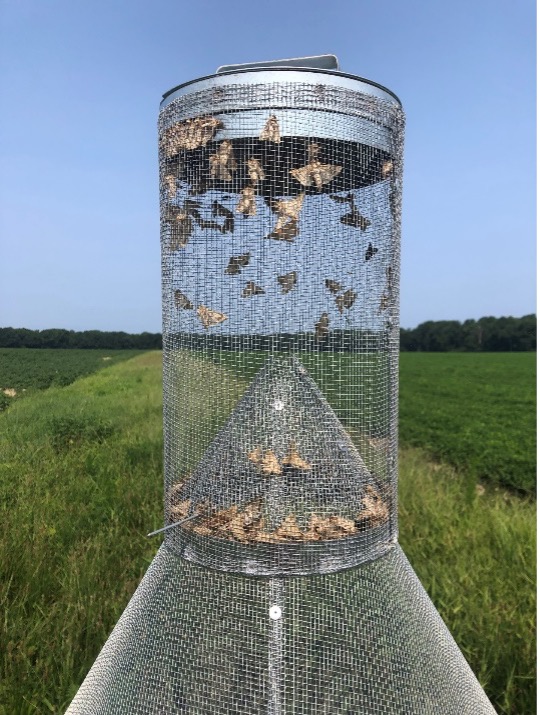
Flexible IPM Tool
Huseth says that as the team develops the traps and analysis, they are accounting for the way pests adapt to their environment.
“This data can help us monitor corn earworms for the development of resistance or changes in population persistence,” he says. “The pest can develop resistance to both Bt technology used in corn and cotton and the insecticides used in many crops.”
This project leverages USDA funding to investigate the potential for shifts in the range of corn earworm populations. Corn earworm pressure is common in southern states where it overwinters, and the pest migrates north during each growing season. Historically it doesn’t overwinter north of 40° latitude, which runs near Trenton, New Jersey; Philadelphia, Pennsylvania; Columbus, Ohio; Indianapolis, Indiana; Springfield, Illinois; St. Joseph, Missouri; and the Kansas-Nebraska state line, unless conditions are mild and dry. If its overwintering zone expands, more farmers will need to understand moth flight patterns to prevent yield losses.
“We are looking ahead to potential developments like this,” Huseth continues. “Our traps are affordable and can be used anywhere. The process of data analysis will also apply in other regions.”
While Huseth and the NCSU team are focusing on corn earworm, they are intentionally developing versatile automated traps and data analysis that can be adapted for other moths and pest complexes.
“By simply changing the pheromone in the trap, it will attract and count a different type of moth,” he says. “The process we are developing to understand and show corn earworm patterns can be repeated using similar data for other pests.”
Huseth believes the automated traps and real-time data will provide a flexible approach to obtaining timely information and risk prediction, which will improve IPM programs.
Published: Aug 1, 2022
The materials on SRIN were funded with checkoff dollars from United Soybean Board and the North Central Soybean Research Program. To find checkoff funded research related to this research highlight or to see other checkoff research projects, please visit the National Soybean Checkoff Research Database.