Research HighlightsAerial Images Show Promise to Support In-Season Management Decisions
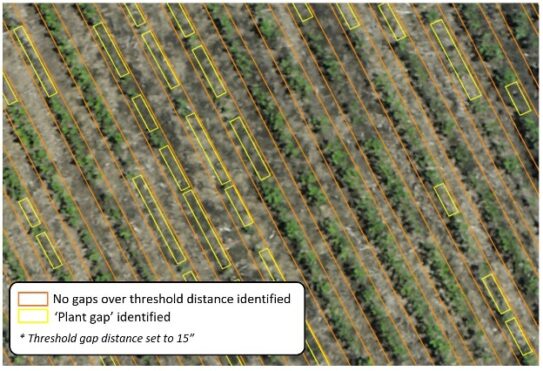
By Laura Temple
Technology continues to transform agriculture. New tools can support farmer profitability, but finding the value in specific technologies can be a challenge.
“Small, unmanned aerial vehicles, commonly called drones, are transforming how aerial imagery is captured and used,” says Rob Austin, Geographic Information Systems (GIS) Extension and precision agriculture specialist with North Carolina State Extension. “Many farmers are interested in this technology but are unsure about the value in this tool today. Farmers want to know if it can help support on-farm decisions that are directly related to yield and profit.”
The North Carolina Soybean Producers Association agrees that farmers need answers to these questions. It is funding research led by Austin to better understand the applications and value of on-farm aerial imagery. His goals for this three-year project are to identify the most promising and profitable uses for current aerial imagery technology in soybean production.
“The technologies that support capturing pictures from above farm fields are rapidly changing,” he explains. “Drones and satellites can now collect more and better detailed pictures than ever, and advances in machine learning and computer vision support new opportunities to process these images into meaningful information. Our challenge is to match and identify the best imaging technology with the applications that derive the most value for farmers. Because drone and satellite imagery are often included as part of a larger package of agronomic services, it can be hard to place a value on the imagery or technology alone.”
Comparing Drone and Satellite Images
During the first year of the research project, Austin worked with farmers and county Extension agents throughout North Carolina to gather a wide variety of on-farm images from both drones and satellite imagery services. He visually and analytically compared these images to evaluate tradeoffs between these technologies.
“Farmers should consider several factors when deciding the type of imagery most helpful to decision-making for their operation,” he says.
He found that drones efficiently gather data in smaller fields, or from specific areas within larger fields. The processed images from drones were much more effective at identifying soybean stress compared to satellite imagery. This was primarily due to the much higher image resolutions achieved by low-flying drones. However, once field size exceeded 200 acres, the rotary-type drones were better suited to scouting smaller areas withing a field and required a more targeted approach to identifying problem areas.
“Drones can only see part of a field at a time, so many overlapping images are collected and stitched together to reveal a complete picture of a field,” Austin explains. “The details are amazing, but the area covered is relatively small when compared to images from satellites.”
New constellations of satellites can provide nearly daily images, and subscription services can provide farmers with near-real-time information from those images. However, the resolution of these images is about 100 times lower than drone images, and cloud cover is an unpredictable factor that can obscure images.
“Drone and satellite imaging technologies are on different ends of the capability spectrum,” he adds. “However, their technical capabilities are moving toward each other and offer potential to complement one another.”
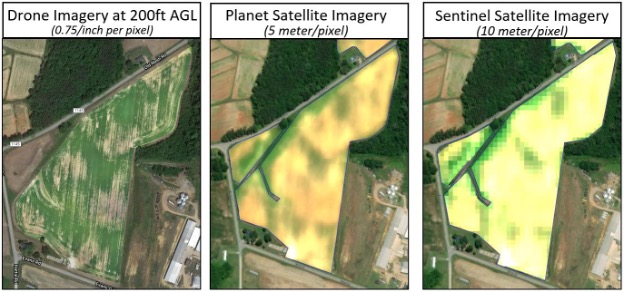
In addition, farmers need to consider the time, hardware and software costs to collect and interpret the images into meaningful data for management decisions. Post-processing of images and analysis can be done either as a subscription service or as a do-it-yourself approach that includes additional hardware and software. Farmers need to weigh their interest in navigating analysis themselves with the convenience and drawbacks of cloud-based mapping solutions for both drone and satellite images.
Practical, Profitable Applications Today
For both types of imagery, Austin pointed out that though soybean stress can be identified in the imagery, on-ground inspection and agricultural knowledge was required to diagnose causes of stress.
“For farmers making decisions that affect the bottom line, they need to catch problems early,” he says. “We found that current drone technology holds a lot of promise for making replant decisions.”
Austin explains that images can now be taken at a resolutions that allow individual plants to be seen and identified soon after emergence. When paired with new machine learning approaches, the images can be used to count the length and number of missing plants within a row, known as a gap analysis, which provides an estimate of emerged soybeans used to estimate stand count and aid in replant decisions.
However, he emphasizes that the relationships between soybean stage, vegetative cover and final yield need to be identified to make sound, profitable decisions about when replants are needed, especially in soybeans, which can branch out to recover from poor emergence.
Research trials in 2022 will help analyze and quantify those relationships. Austin has planned both plot-scale and field-scale strip trials with historically poor soybean emergence. Early-season images of these fields will be analyzed for gaps in stand and compared to yield at the end of the season to begin developing indicators and recommendations based on stand “gaps” that will help farmers decide when a section of a field is worth replanting.
“Although we didn’t focus on weed detection and identification in 2021, we gathered images and performed some preliminary analysis that showed promise,” Austin continues. “We are interested in whether problematic weeds like Palmer amaranth, ragweed and sicklepod can not only be identified within a field of soybeans, but also if we can differentiate and count different so weed control may also be a practical application for aerial imagery.”
To further explore this potential application, they plan to collect images over a herbicide trial set up to create different levels of weed pressure by species. His team also plans to artificially simulate different weed outbreaks by placing weeds grown in pots at different sizes and stages throughout an established soybean field. These fields will be flown using less-expensive, consumer-grade drone equipment, as well as more costly and advanced equipment that collects multispectral images. This work will confirm the ability to scout for these problem weeds from the air, as well as the necessary equipment and costs.
Both the stand gap analysis and weed identification research will help farmers identify applications and realize actual value from current aerial imaging technology. Understanding how best to use these tools today will allow farmers to benefit more quickly as technology advances to support more applications in the future.
Published: Jun 6, 2022
The materials on SRIN were funded with checkoff dollars from United Soybean Board and the North Central Soybean Research Program. To find checkoff funded research related to this research highlight or to see other checkoff research projects, please visit the National Soybean Checkoff Research Database.